The digitization of histopathology slides has transformed how we diagnose and study disease. With the help of powerful deep learning algorithms, computers can now analyze tumor slides at an unprecedented scale. However, this leap in technology brings with it a surprising and concerning risk: the potential to trace digital histology images back to individual patients, even if their personal data has been removed.
In a recent study [1], our team explored this risk. We asked a simple but important question: can an AI be trained to recognize which patient a histopathology image came from, even after the data has been anonymized?
The Context: Why This Matters
Whole slide images, or WSIs, are routinely used in cancer diagnosis and research. When large WSI datasets are shared publicly to support AI development, they are carefully anonymized. All metadata that could identify a patient is removed.
However, modern deep learning models are incredibly good at picking up subtle patterns. Previous studies have shown that even seemingly generic medical images like chest X-rays[2] or MRIs of the knee[3] or head[4] contain enough information to recognize individuals. As our group has published many histopathology dataset over the years, the question whether something similar might be possible using WSIs can to us quite naturally.
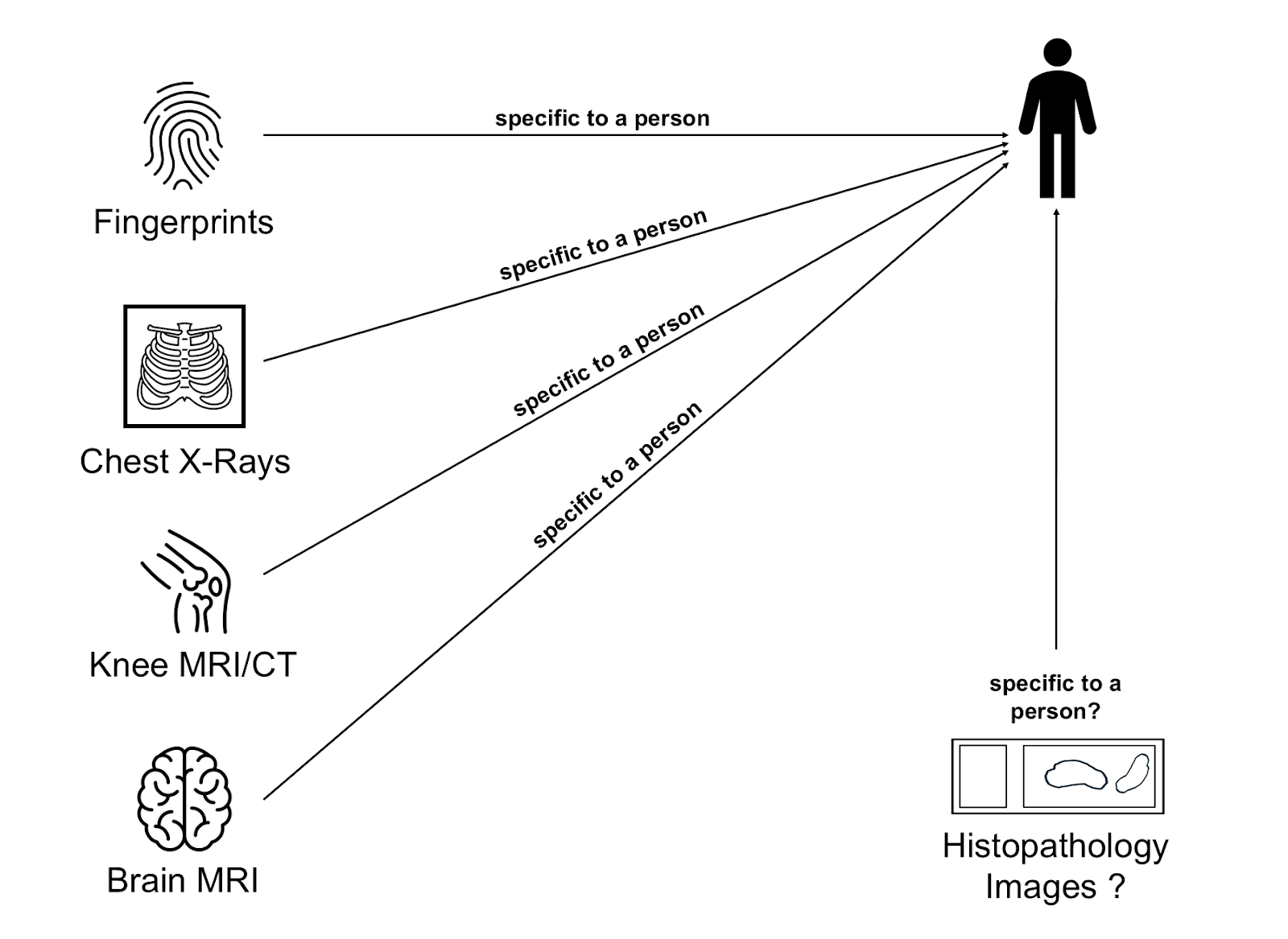
The Study: Can Histology Slides Reveal Identity?
We set out to test whether AI models could re-identify patients by analyzing the microscopic appearance of their tissue. Specifically, we wanted to know:
- Can patients be re-identified from anonymized histopathology images?
- Can this still happen if the tissue samples were taken at different points in time?
To answer these questions, we used three large datasets: two public lung cancer datasets (LUAD and LSCC from TCIA) and one in-house dataset of brain tumor tissue (meningiomas). In each case, we used only images from patients with at least two slides available. This allowed us to train and test our models on different samples from the same individuals.
How the Models Work
We used different multiple instance learning architectures together with various feature extractors. The re-identification problem was framed as a classification task, where each patient was associated with a unique class.
Additionally, we conducted experiments to investigate the influence of stain-related cues on the models’ performance. In our journal article, we also discussed other covariant factors that might influence model accuracy.
Key Findings: Recognition Is Possible — with Limitations
We found that re-identification is indeed possible with high accuracy (up to 80%) when the training data includes slides from the same resection. So to answer the first question: yes, re-identification from histopathology images is possible, and it can be highly accurate.
However, when the models were trained and tested on slides taken at different points in time, their performance dropped significantly. Despite this, the accuracy still remained higher than what would be expected by random guessing. We concluded that while re-identification is generally not reliable across different time points, the cases where it still worked may be due to morphological similarities between a primary tumor and a regrowth following an incomplete resection.
Key Takeaways
Re-identification is possible with high accuracy, but mainly when performed on slides from the same resection. This suggests that our models were more likely identifying tumors rather than patients. Nonetheless, this finding has implications for patient privacy.
If this makes you worried that you can no longer share your WSIs, don’t be. We developed a risk assessment framework that can help estimate the potential privacy risks to patients before data is published. All about that can be found in our paper.
Comments are closed