Funded by: DFG (Deutsche Forschungsgemeinschaft) / FWF (Österreichischer Wissenschaftsfonds)
Neoplasia, a common cause of death in both animals and humans, requires treatment decisions based on pathological examination of tumor specimens. Histological analysis, particularly mitotic count (MC), plays a crucial role in prognostication. However, our research highlights significant challenges, including heterogeneity in MC distribution throughout histological slides, observer-dependent variability, morphological complexities, and technical limitations in mitotic figure detection. These factors contribute to poor agreement among trained pathologists, with published κ values between 0.08 and 0.77, posing a risk of incorrect prognostication and therapeutic decisions (Bertram et al., 2018; Donovan et al., 2020).
Our research shows that computer-aided image analysis using machine/deep learning techniques excels in histopathologic classification tasks on H&E-stained slides, surpassing human observers with ample data (Bertram et al., 2022; Bertram 2019a). We’ve developed large-scale, high-quality labeled datasets, and our algorithm has proven to enhance pathologists‘ performance significantly (Bertram et al., 2022). This advancement suggests a more reliable tumor prognostication using the cost-effective H&E-stained slides (Villani et al., 2016; Tracht, Zhang, and Peker, 2017).
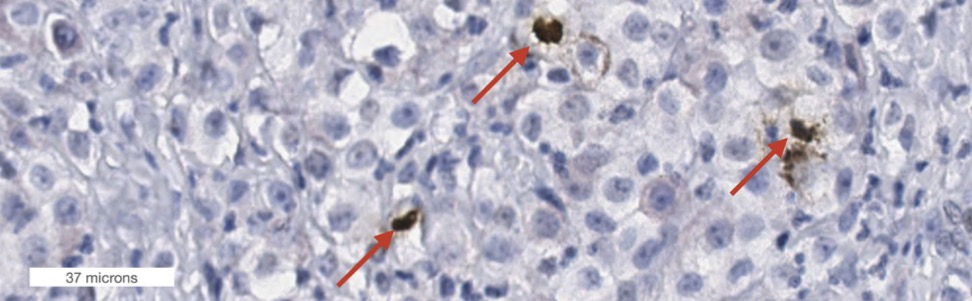
The need for a multi-domain reference whole slide image data set as open data
Successful implementation of deep learning-based, data-driven methods hinges on access to representative large-scale datasets. Image domains in histopathology, encompassing staining variations, species, tissue types, and digitization devices, contribute to dataset variability. Different regions within histological sections may contain diverse tissue components and artifacts, potentially rendering selected regions of interest (ROI) non-representative (Aubreville et al., 2020b; Bertram et al., 2019a). This variation leads to a domain shift in data distribution, impacting algorithm performance significantly (Aubreville et al., 2021b; Stacke et al., 2021). Addressing this challenge through domain adaptation approaches is crucial in computational histopathology, and while progress has been made, a persistent domain gap impedes the development of robust software solutions applicable to the full spectrum of biological and imaging variations in digitized histological tumor sections across different tissue types, image locations, laboratories, species, and scanners (Aubreville et al., 2020a; Stacke et al., 2021).
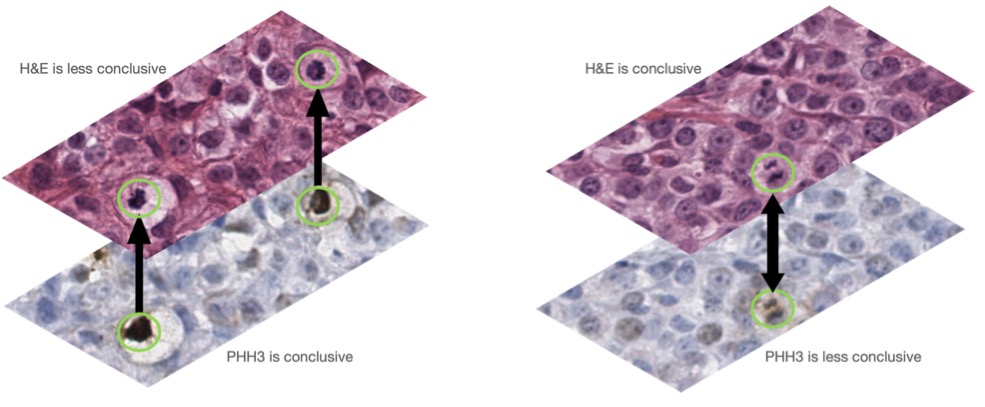
Co-registered H&E and PHH3 stains. The left image shows mitotic figures in an early phase (PHHE-positive) and the right panels show a mitotic figure in a late phase (PHHE-negative).