This project aims to enhance the diagnosis of tissue samples, particularly in determining tumor malignancy, through the use of artificial intelligence (AI) methods on digitized tissue sections. While AI has demonstrated improved diagnostic reproducibility, speed, and accuracy, challenges arise in cases with borderline malignancy due to multiple relevant focus planes in tissue sections. Unlike traditional microscopy, digital microscopy captures only one focus setting, leading to the loss of diagnostic information. This issue is prominent in histopathology and further exacerbated in the examination of detached tissue cells (cytopathology). To address these limitations, the project explores three key areas. First, in collaboration with ZEISS Research Microscopy Solutions in Munich, the project aims to robustly detect focus offsets in two-dimensional images, triggering partial re-digitization when the offset exceeds specified tolerances. The second part, in partnership with Tribun Health in Paris, focuses on predicting all diagnostically relevant focus planes from two-dimensional images and recognizing pathological patterns in multi z-stacks. These efforts aim to reduce economic factors and facilitate diagnostic applications in the medical field.
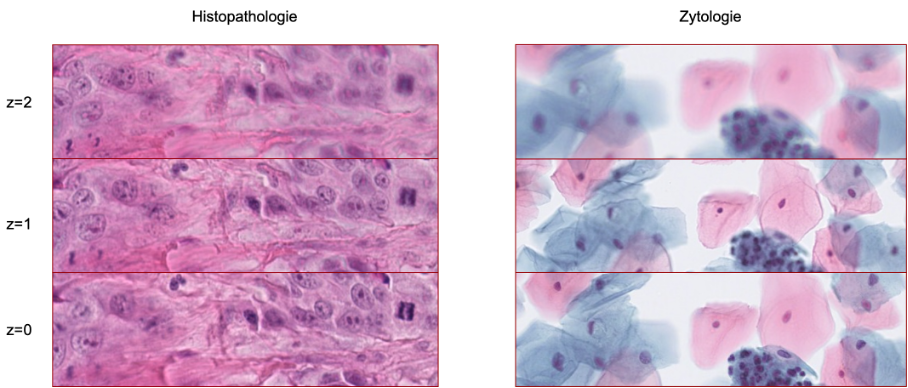