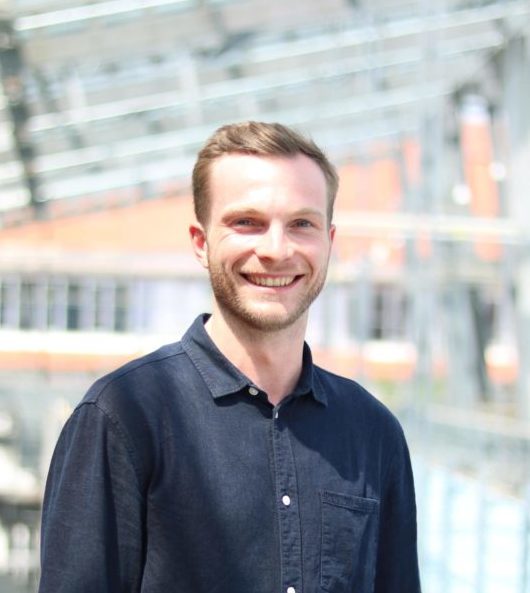
Jonas Ammeling
I am a research assistant and PhD student at the Technical University of Applied Sciences Ingolstadt. I received my Master’s degree in Statistics & Data Science from Leiden University in the Netherlands. My current work focuses on the development of deep learning applications in digital pathology and radiology. I am particularly interested in object detection methods, self-supervised learning, more user-centric approaches, and multimodal vision-language processing.
Publications:
2025
An interdisciplinary perspective on AI-supported decision making in medicine Journal Article
In: Technology in Society, vol. 81, pp. 102791, 2025, ISSN: 0160791X.
“When Two Wrongs Don’t Make a Right” – Examining Confirmation Bias and the Role of Time Pressure During Human-AI Collaboration in Computational Pathology Proceedings Article
In: Proceedings of the 2025 CHI Conference on Human Factors in Computing Systems, pp. 1–18, ACM, Yokohama Japan, 2025, ISBN: 9798400713941.
Automation Bias in AI-assisted Medical Decision-making under Time Pressure in Computational Pathology Book Section
In: Palm, Christoph; Breininger, Katharina; Deserno, Thomas; Handels, Heinz; Maier, Andreas; Maier-Hein, Klaus H.; Tolxdorff, Thomas M. (Ed.): Bildverarbeitung für die Medizin 2025, pp. 129–134, Springer Fachmedien Wiesbaden, Wiesbaden, 2025, ISBN: 9783658474218 9783658474225.
In: Palm, Christoph; Breininger, Katharina; Deserno, Thomas; Handels, Heinz; Maier, Andreas; Maier-Hein, Klaus H.; Tolxdorff, Thomas M. (Ed.): Bildverarbeitung für die Medizin 2025, pp. 63–68, Springer Fachmedien Wiesbaden, Wiesbaden, 2025, ISBN: 9783658474218 9783658474225.
Histologic Dataset of Normal and Atypical Mitotic Figures on Human Breast Cancer (AMi-Br) Book Section
In: Palm, Christoph; Breininger, Katharina; Deserno, Thomas; Handels, Heinz; Maier, Andreas; Maier-Hein, Klaus H.; Tolxdorff, Thomas M. (Ed.): Bildverarbeitung für die Medizin 2025, pp. 113–118, Springer Fachmedien Wiesbaden, Wiesbaden, 2025, ISBN: 9783658474218 9783658474225.
Comprehensive Dataset of Coarse Tumor Annotations for The Cancer Genome Atlas Breast Invasive Carcinoma Book Section
In: Palm, Christoph; Breininger, Katharina; Deserno, Thomas; Handels, Heinz; Maier, Andreas; Maier-Hein, Klaus H.; Tolxdorff, Thomas M. (Ed.): Bildverarbeitung für die Medizin 2025, pp. 260–265, Springer Fachmedien Wiesbaden, Wiesbaden, 2025, ISBN: 9783658474218 9783658474225.
Investigation of Class Separability within Object Detection Models in Histopathology Journal Article
In: IEEE Transactions on Medical Imaging, pp. 1–1, 2025, ISSN: 0278-0062, 1558-254X.
2024
An Interdisciplinary Perspective on AI-Supported Decision Making in Medicine Journal Article
In: Technology in Society, pp. 102791, 2024, ISSN: 0160791X.
Information mismatch in PHH3-assisted mitosis annotation leads to interpretation shifts in H&E slide analysis Journal Article
In: Scientific Reports, vol. 14, no. 1, pp. 26273, 2024, ISSN: 2045-2322.
Prediction of tumor board procedural recommendations using large language models Journal Article
In: European Archives of Oto-Rhino-Laryngology, 2024, ISSN: 1434-4726.
Re-identification from histopathology images Journal Article
In: Medical Image Analysis, pp. 103335, 2024, ISSN: 13618415.
Model-based Cleaning of the QUILT-1M Pathology Dataset for Text-Conditional Image Synthesis Proceedings Article
In: Medical Imaging with Deep Learning, Paris, France, 2024.
In: Medical Image Analysis, vol. 94, pp. 103155, 2024, ISSN: 13618415.
Perceived responsibility in AI-supported medicine Journal Article
In: AI & SOCIETY, 2024, ISSN: 0951-5666, 1435-5655.
Perceived responsibility in AI-supported medicine Journal Article
In: AI & SOCIETY, 2024, ISSN: 0951-5666, 1435-5655.
Few Shot Learning for the Classification of Confocal Laser Endomicroscopy Images of Head and Neck Tumors Book Section
In: Maier, Andreas; Deserno, Thomas M.; Handels, Heinz; Maier-Hein, Klaus; Palm, Christoph; Tolxdorff, Thomas (Ed.): Bildverarbeitung für die Medizin 2024, pp. 143–148, Springer Fachmedien Wiesbaden, Wiesbaden, 2024, ISBN: 9783658440367 9783658440374.
Automated Mitotic Index Calculation via Deep Learning and Immunohistochemistry Book Section
In: Maier, Andreas; Deserno, Thomas M.; Handels, Heinz; Maier-Hein, Klaus; Palm, Christoph; Tolxdorff, Thomas (Ed.): Bildverarbeitung für die Medizin 2024, pp. 123–128, Springer Fachmedien Wiesbaden, Wiesbaden, 2024, ISBN: 9783658440367 9783658440374.
Assessment of Scanner Domain Shifts in Deep Multiple Instance Learning Book Section
In: Maier, Andreas; Deserno, Thomas M.; Handels, Heinz; Maier-Hein, Klaus; Palm, Christoph; Tolxdorff, Thomas (Ed.): Bildverarbeitung für die Medizin 2024, pp. 137–142, Springer Fachmedien Wiesbaden, Wiesbaden, 2024, ISBN: 9783658440367 9783658440374.
2023
Appealing but Potentially Biasing – Investigation of the Visual Representation of Segmentation Predictions by AI Recommender Systems for Medical Decision Making Proceedings Article
In: MuC ’23: Mensch und Computer 2023, pp. 330–335, ACM, Rapperswil Switzerland, 2023, ISBN: 9798400707711.
A comprehensive multi-domain dataset for mitotic figure detection Journal Article
In: Scientific Data, vol. 10, no. 1, pp. 484, 2023, ISSN: 2052-4463.
Attention-based Multiple Instance Learning for Survival Prediction on Lung Cancer Tissue Microarrays Book Section
In: Deserno, Thomas M.; Handels, Heinz; Maier, Andreas; Maier-Hein, Klaus; Palm, Christoph; Tolxdorff, Thomas (Ed.): Bildverarbeitung für die Medizin 2023, pp. 220–225, Springer Fachmedien Wiesbaden, Wiesbaden, 2023, ISBN: 978-3-658-41656-0 978-3-658-41657-7, (Series Title: Informatik aktuell).
Deep Learning-based Subtyping of Atypical and Normal Mitoses using a Hierarchical Anchor-free Object Detector Book Section
In: Deserno, Thomas M.; Handels, Heinz; Maier, Andreas; Maier-Hein, Klaus; Palm, Christoph; Tolxdorff, Thomas (Ed.): Bildverarbeitung für die Medizin 2023, pp. 189–195, Springer Fachmedien Wiesbaden, Wiesbaden, 2023, ISBN: 978-3-658-41656-0 978-3-658-41657-7, (Series Title: Informatik aktuell).
Deep Learning-based Automatic Assessment of AgNOR-scores in Histopathology Images Book Section
In: Deserno, Thomas M.; Handels, Heinz; Maier, Andreas; Maier-Hein, Klaus; Palm, Christoph; Tolxdorff, Thomas (Ed.): Bildverarbeitung für die Medizin 2023, pp. 226–231, Springer Fachmedien Wiesbaden, Wiesbaden, 2023, ISBN: 978-3-658-41656-0 978-3-658-41657-7, (Series Title: Informatik aktuell).
Reference Algorithms for the Mitosis Domain Generalization (MIDOG) 2022 Challenge Book Section
In: Sheng, Bin; Aubreville, Marc (Ed.): Mitosis Domain Generalization and Diabetic Retinopathy Analysis, vol. 13597, pp. 201–205, Springer Nature Switzerland, Cham, 2023, ISBN: 978-3-031-33657-7 978-3-031-33658-4, (Series Title: Lecture Notes in Computer Science).