Counting mitotic figures (MFs) under a microscope is a crucial component for tumor diagnosis and therapy planning. Our research group has been dedicated to automating this task through computer-assisted MF identification algorithms, significantly enhancing efficiency and accuracy.
Advancing Research with Comprehensive Datasets
Collaborating closely with pathologists, we have developed and published multiple MF datasets to fuel research in this area. One notable dataset, MITOC_WSI_CMC [1], includes 21 whole slide images (WSIs) of canine mammary carcinoma (CMC), featuring 13,907 MFs and 36,379 hard negatives. Creating such datasets is no small feat: pathologists meticulously examine every single cell in each WSI to determine its division status. To ensure accuracy and minimize subjectivity, we asked at least two experts to reach a consensus on each annotated MF.
Selective Annotation: Maximizing Efficiency
To optimize the efforts of our pathologists, we have been focusing on selective annotation. It targets only the most informative regions of an image for expert annotation, leaving less critical areas unlabeled. For our case, the key is identifying regions rich in MFs, which provide valuable training data for supervising the MF identification models. But how can we pinpoint these regions without a medical background?
Introducing: Prototype Sampling
In our recent MICCAI24 paper, we introduced a novel method called “prototype sampling” to address this challenge. We utilize histopathology image-caption databases to recognize MF prototypes, which then help identify annotation regions that resemble these prototypes. Two databases were used in our study:
- ARCH [2] : Contains figures and captions from PubMed articles and textbooks.
- OpenPath [3] : Includes Tweets where pathologists discussed cases.
These resources offer a diverse collection of images showcasing both typical and ambiguous disease aspects, with variations in staining material and case origin. See an example below.
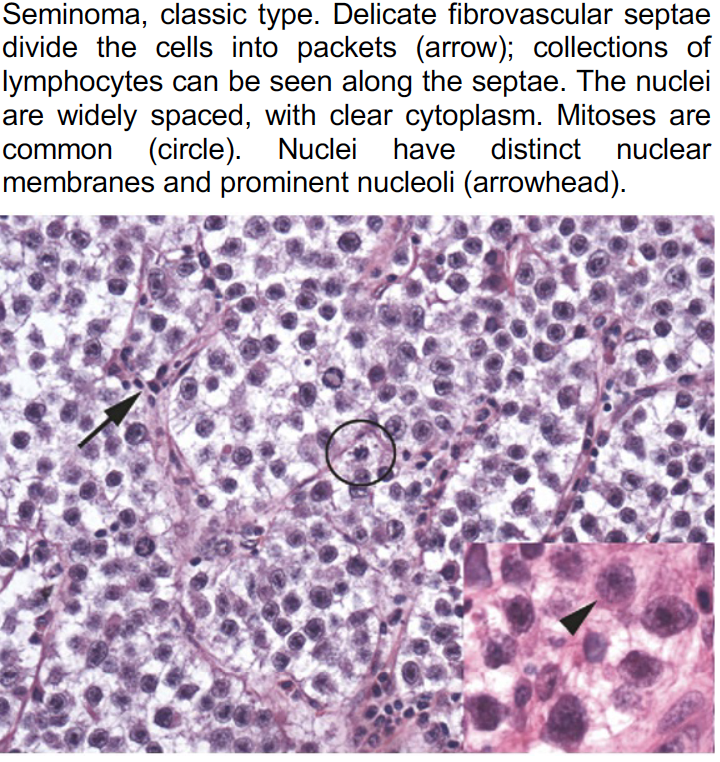
Prototype sampling: How it works
We employed two techniques to identify MF prototypes from image-caption databases: keyword search and text-to-image retrieval. The WSI is then divided into small patches, each containing only one or a few cells. By calculating the similarity of each patch to the MF prototypes, we generate a similarity map highlighting areas likely to contain MFs. Regions with the highest accumulated similarities are selected for annotation.
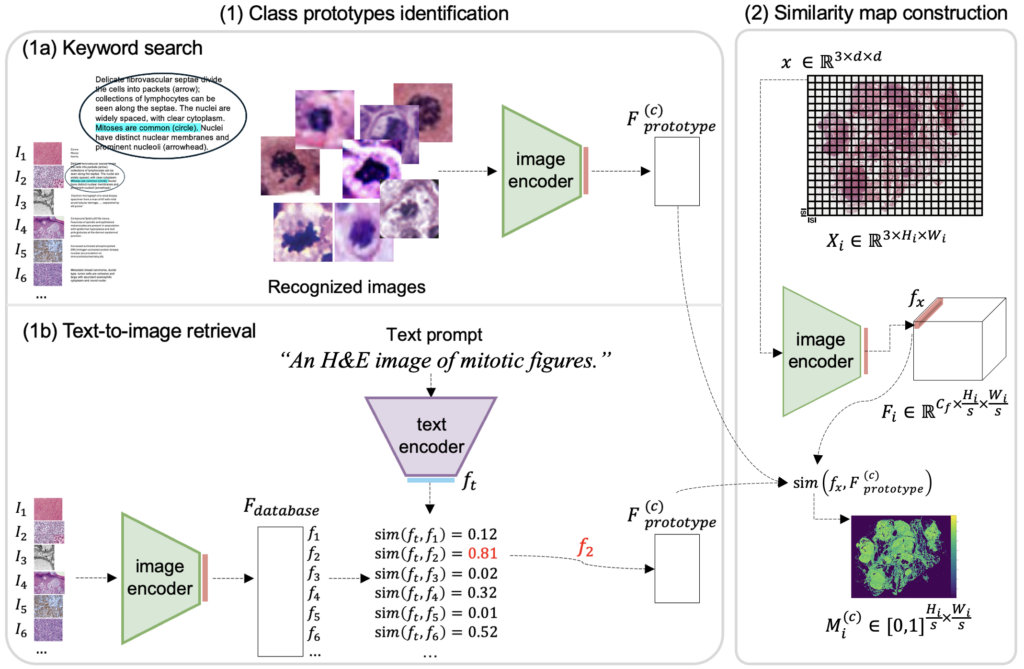
Results: How well prototype sampling works
Our findings show that prototype sampling identifies regions with more MFs than other compared methods, resulting in better-trained models. In particular, annotating just 18% of the area using our method nearly matches the performance of the fully annotated dataset. Our approach significantly reduces the annotation workload for our pathologists.
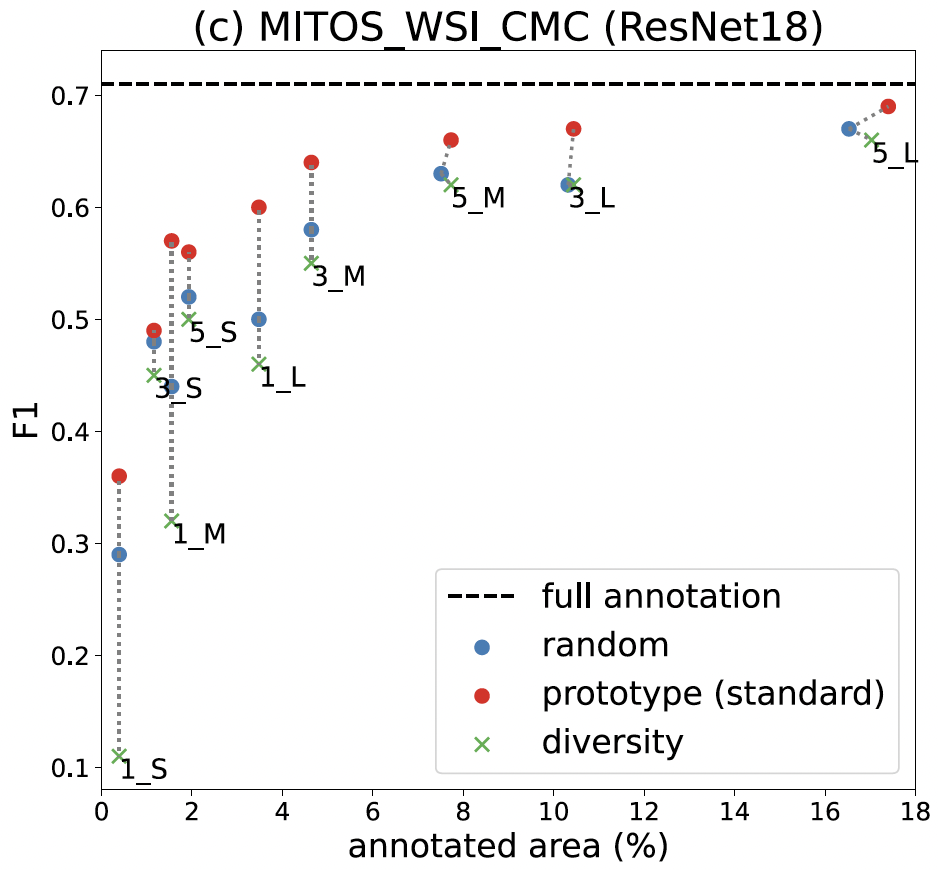
Beyond mitotic figures: Prototype sampling for other tasks
We also test the effectiveness of prototype sampling for simplifying segmentation mask acquisition and examine the robustness of our approach on image captioning databases of varying quality and with different annotation budgets. For more technical details and insights into our research, explore our published paper!
[1] Aubreville, M., Bertram, C.A., Donovan, T.A., Marzahl, C., Maier, A., Klopfleisch, R.: A completely annotated whole slide image dataset of canine breast cancer to aid human breast cancer research. Scientific data 7(1), 417 (2020)
[2] Gamper, J., Rajpoot, N.: Multiple instance captioning: Learning representations from histopathology textbooks and articles. In CVPR (2021)
[3] Huang, Z., Bianchi, F., Yuksekgonul, M., Montine, T., Zou, J.: Leveraging medical twitter to build a visual–language foundation model for pathology ai. bioRxiv pp.2023–03 (2023)
Comments are closed