The overall objective of this research proposal is to develop and evaluate novel methods for the diagnosis and treatment of sinonasal tract tumors using CLE, with a focus on improving patient outcomes through enhanced visualization and decision support.
The diagnosis and treatment of sinonasal tract tumors pose significant challenges due to their rarity, complex anatomy, and limited visibility during surgical procedures. Traditional imaging techniques, such as computed tomography (CT) scans and magnetic resonance imaging (MRI), have limitations in providing detailed information about the tumor’s size, shape, location, and relationship with surrounding structures.
To address these challenges, researchers have been exploring the use of Confocal Laser Endoscopy (CLE) as a novel diagnostic tool. CLE involves using endoscopic instruments to visualize the inside of the nasal cavity and sinuses, allowing for more precise and minimally invasive diagnosis and treatment of tumors. However, the development of effective CLE systems requires significant advances in image processing, machine learning, and user interface design.
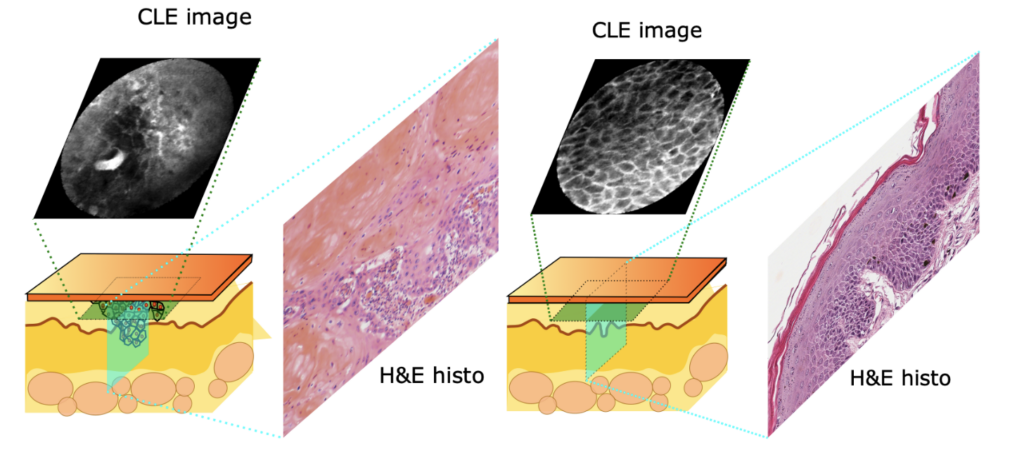
Our project, which is funded by the German Research Foundation (Deutsche Forschungsgemeinschaft, DFG) and conducted together with the ENT clinic of the university hospital of the University of Würzburg (Principal Investigator: PD Dr. Miguel Gonçalves) aims to address these challenges by developing a novel CLE system that integrates advanced image processing techniques, robust machine learning algorithms, and intuitive user interfaces. The proposed system will be evaluated for its performance in diagnosing and treating sinonasal tract tumors, with a focus on improving patient outcomes through enhanced visualization and decision support.
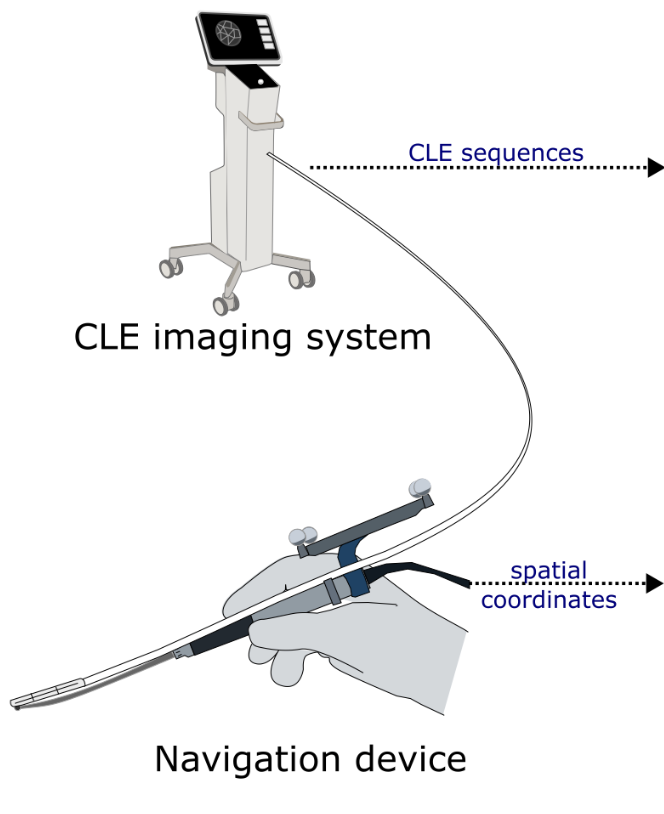
In particular, we aim to co-register CLE information with the patient’s anatomy. For this, we use an off-the-shelve navigation device, and bundle it with the fine fibre bundle of the CLE device. This, together with a prior registration on the patient’s landmarks, allows to do a post-hoc analysis of tissue organization within the patient’s anatomy.
The complex patterns acquired from the CLE device, however, are challenging to interpret, and the projection onto the anatomical situation in 3D is a challenge for the experts. This is why we use machine learning models to evaluate the malignancy of the CLE frames, condensing the information significantly, and allowing for a visualization in the next step.
Ultimately, we want to provide a virtual endomicroscopy using 3D visualization techniques, where tumorous areas are visualized. We aim to investigate the potential of pre-surgical or post-surgical analysis for improving patient outcome using these techniques.
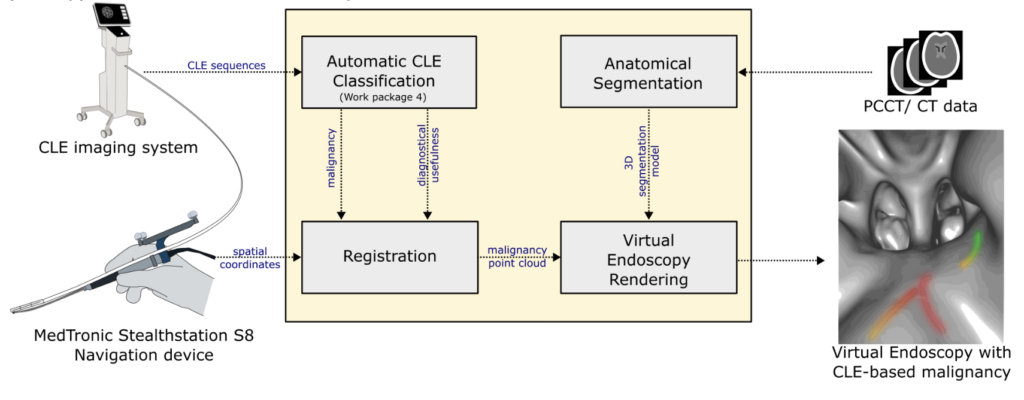
The proposed research has the potential to revolutionize the diagnosis and treatment of sinonasal tract tumors, enabling clinicians to provide more accurate diagnoses, effective treatments, and improved patient outcomes.
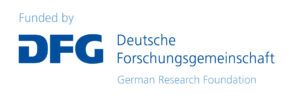