We have another paper on MIDL 2024, and we want to briefly give you an overview here. It is about the detection of mitotic figures (cells undergoing cell division), which is really important in the grading of many cancer types, including breast cancer. The paper was a collaboration with Rutger H.J. Fick (and of course, our friend and veterinary pathologist Christof Bertram), and it included two main ideas:
- Since whole slide scanners produce a quite strong domain shift – wouldn’t it be a good idea in the creation of a really robust mitosis detector to just scan the same physical slides with multiple scanners to make the deep learning-based detector more robust (a kind of real-world image augmentation)?
- Since multi-task learning was already proven successful in deep learning, given a suitable secondary task, could subtyping of mitosis into typical and atypical mitosis be such a task that helps the detection itself?
If you now say: Wait a minute – I just learnt what mitosis is, and now you are talking about typical and atypical, then let me briefly explain this to you. Some mitotic figures look really not the way they should look, and it is suspected that this is linked to a severe degradation of cell replication, and the presence of those weirdly looking dividing cells could be a sign of malignancy by itself. So this is why this is so relevant. We dug into this topic a while back in a BVM paper.
Rescanning a canine breast cancer dataset
A while back, we published a whole slide image (WSI) dataset of canine breast cancer in Scientific Data. Whole slide image annotations are a lot of work – and I am so grateful that our crazy research friends from pathology (Christof Bertram, Robert Klopfleisch and Taryn Donovan) did not fear the effort. Together we were able to create a dataset of 21 WSIs. This does not sound like a lot – at the end it’s only 21 cases, right? But these 21 WSIs have some 14 thousand individual annotations, all generated as the consensus of three experts. Here is an example image:
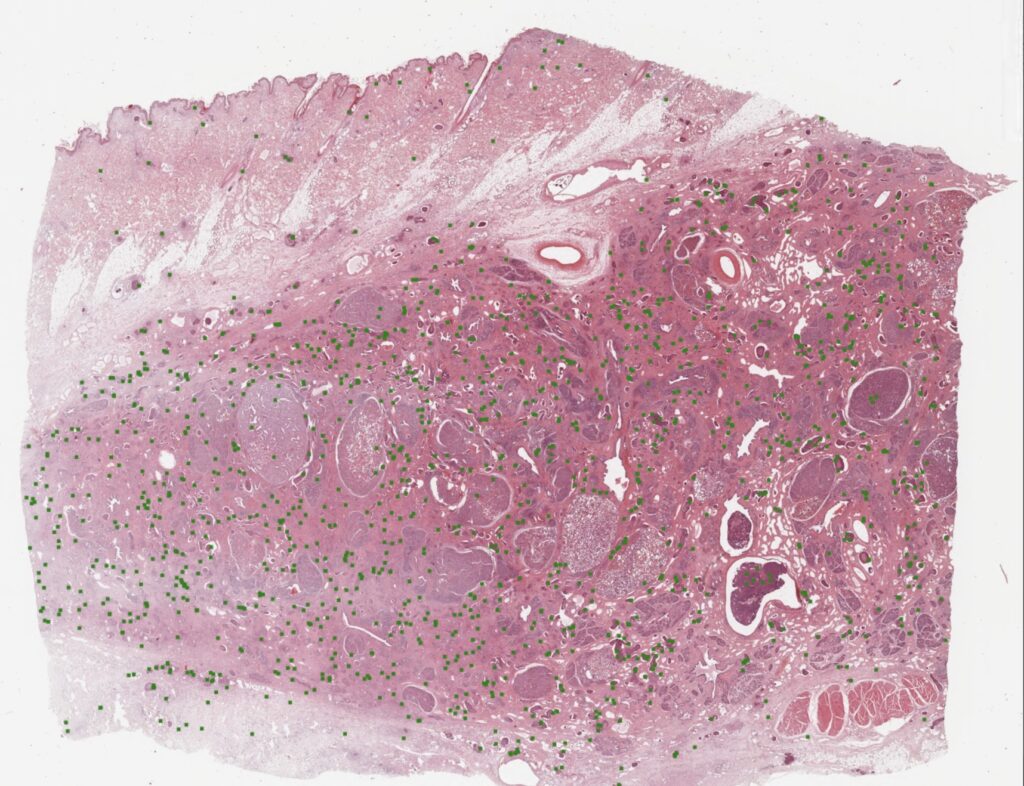
The really cool thing about this, is that our pathologists annotated all areas of the WSIs, also including the ones that are often not used for MC determination (necrotic areas, inflammatory areas, etc…). This real-world data diversity makes our detectors really robust when applied on WSIs.
So, we then went ahead and digitized the samples with a bunch of (7, to be exact) scanners and registered the images:
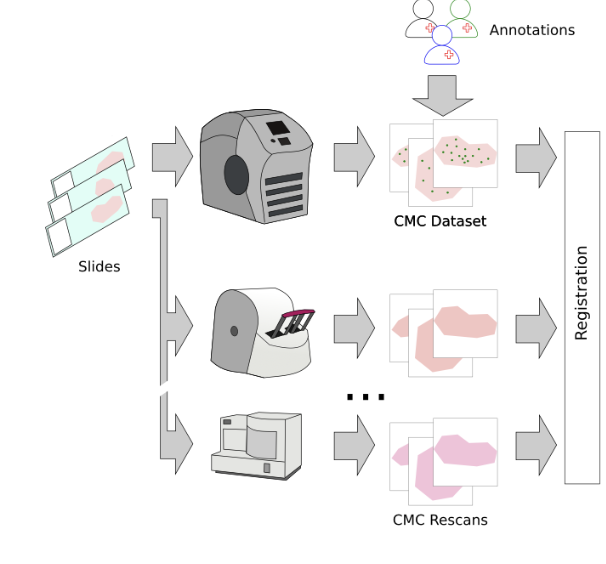
So far, so good. The problem that then occurred was that the annotated objects on the rescanned glass slides were partially out of focus:
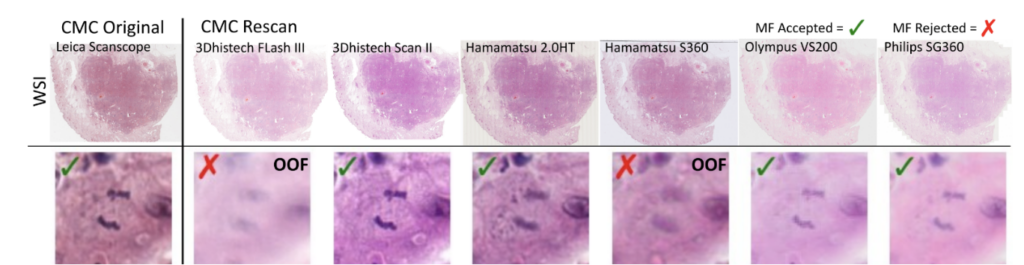
This introduces a noisy label problem. The simple but well-working idea for this was that we performed an ensemble-based classification of the mitotic figure objects. If they were unsure, we removed them. This also means that we removed them from the image:
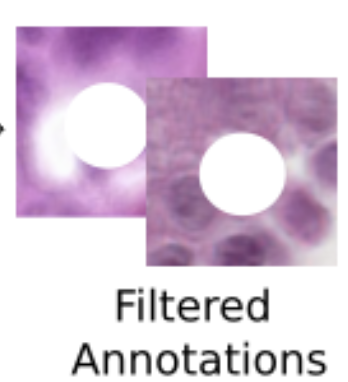
As last step, we performed a second-stage classification that differentiated mitotic figures from similarly looking objects (imposters/hard negatives) and that classified them into typical and atypical MF. We threw in some annotated areas from a diverse set of animal tumors for increased robustness. In total, our pipeline looks like this:
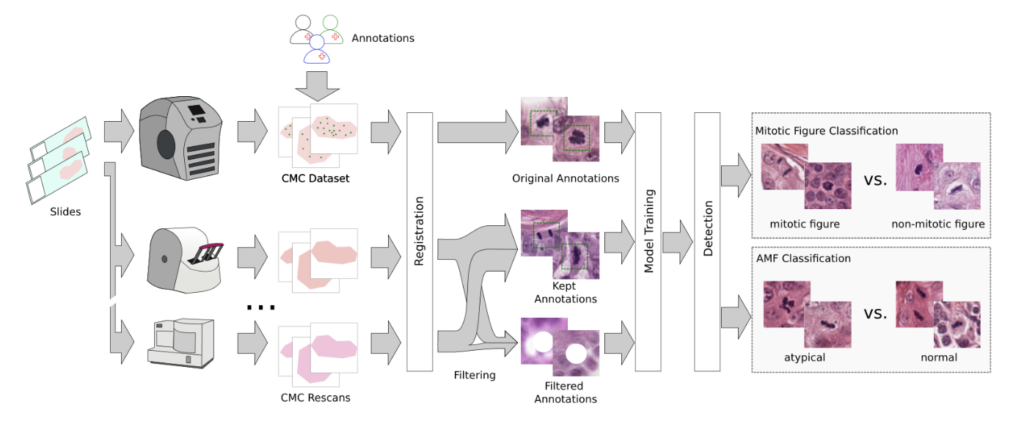
Our results
We tested on the multi-tumor/multi-species MIDOG 2022 training set as our hold out dataset. Let us have a brief look at the performance:
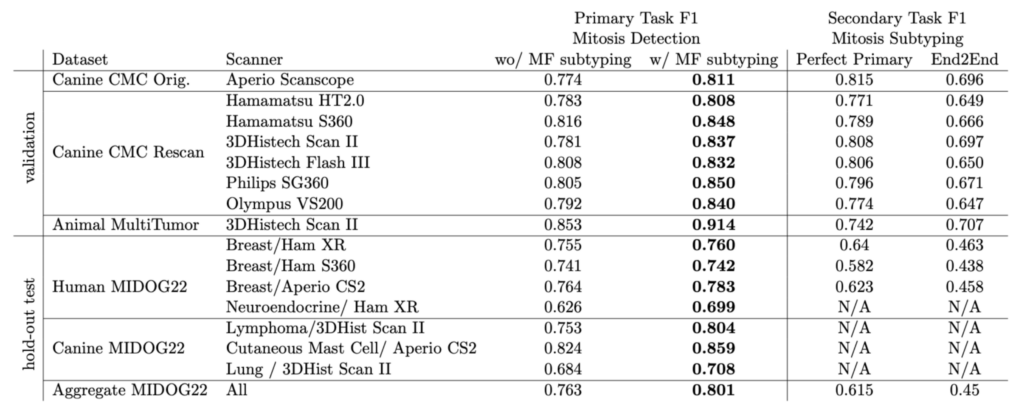
The overall F1 score on the MIDOG dataset was really remarkable, and moreover we saw a compelling effect of the atypical subtyping. In fact, model with atypical subtyping outperformed the model without on all domains.
Take home from this
We have to state that our approach is not very fancy – it just followed the idea of having a good data diversity and high annotation quality. This, however, yielded a very robust and high-quality detector, which works pretty great on human breast cancer, and it was solely trained on animal specimen. Another step towards applying this in clinical routine!
Comments are closed