We will be representing our research again this year at Medical Imaging with Deep Learning (MIDL), to be held in Paris July 3-5th. Here’s a quick summary of one of the research works that we will be presenting there:
Cleaning large online datasets for improved text-to-image in pathology
Large and diverse clinical data corpora for pathology are currently unfortunately not available openly for the research community. While some institutions can utilize their own archives, this is unfortunately not true for the majority of groups that work on computer vision problems in pathology. To counteract this problem, the Ikezogwo et al. have published a dataset purely made from free online resources (youtube, X twitter, etc..) at NeurIPS 2023. They were able to show that this dataset is well-suited for pretraining of networks.
However, if one wants to use the dataset (named QUILT-1M) also for text-to-image tasks, there arises a problem very quickly: The dataset is just littered with image impurities. This is nicely demonstrable when fine-tuning a Stable Diffusion model with it:
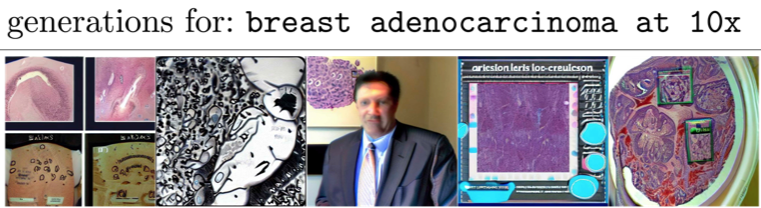
The images above were not even cherry-picked – it was just 5 consecutive generations of the model for the prompt “breast adenocarcinoma at 10x”. This is certainly not what we were hoping for, but it’s also very clear what happened here: The generations are a reflection of the dataset itself.
We then cleaned the dataset using a semi-automatic pipeline (details to be found in the paper) with two steps, and fine-tuned the same model with it. The results differed strongly:
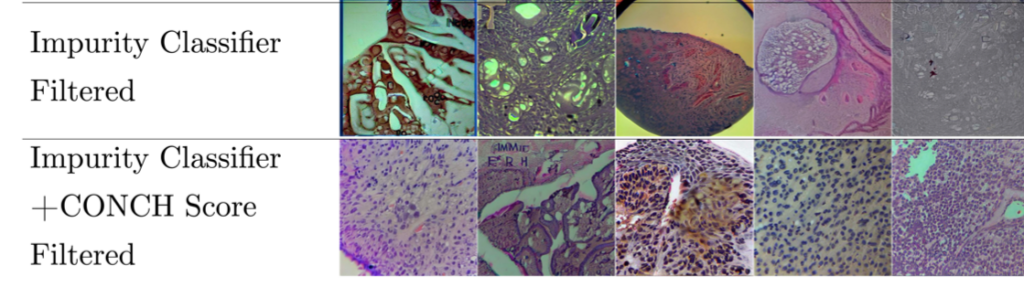
While these were also not perfect, it’s still notable how much more aligned with the expectations these are. This was also confirmed by the metrics that we calculated. All in all, a very straight-forward approach, but it worked 🙂
Comments are closed