Detection of Atypical Mitotic Figures [BVM2023 Talk]
TLDR: Watch our youtube-video at the end of the post.
In our recent work, we dig into the topic of not only detecting mitotic figures in histopathology slides but also of classifying them into typical and atypical cases. As shown by Oshashi et al. in 2018 and Lashen et al. in 2022 the presence and rate of atypical mitotic figures is an independent prognostic factor for malignancy.
We can subdivide mitosis into several phases that occur in a typical cell division process. Besides that, especially in tumors, we find atypical mitotic figures that are representing a defunctive mitotic process.
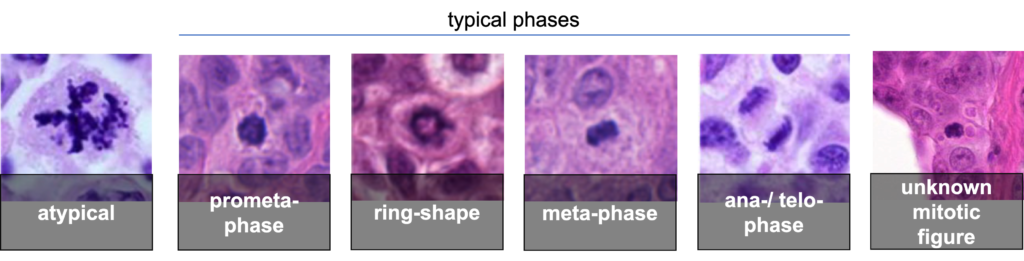
When labeling the mitotic figures of the TUPAC16 and MIDOG21 dataset, we found that there is a significant disagreement between experts:
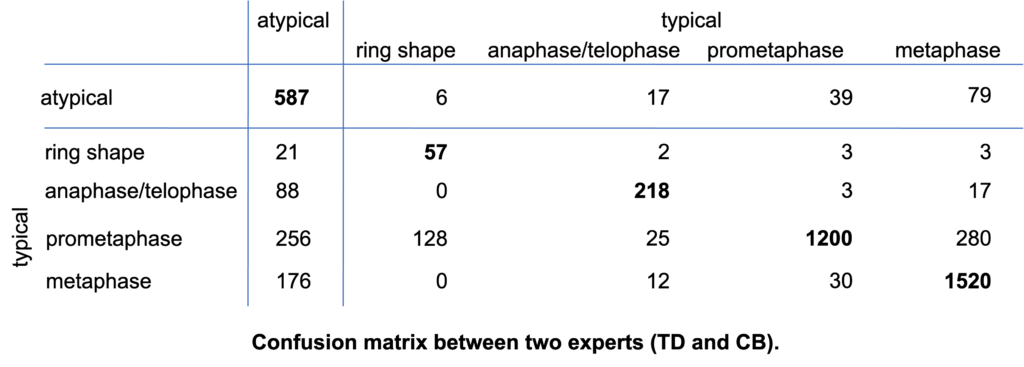
This disagreement complicates our object detection problem. While we want to make sure that we detect each and every mitotic figure, we also want to classify them into being atypical or typical, and also into the different phases of mitosis. On top of that, we have cells that have an unknown subclass state, since the experts did not agree. Phrasing this as a 6-class classification problem, however, introduces the problem that the unknown class is not disjunctive from the other classes, and even the atypical and the different phases of the typical mitosis are not 100% disjunctive.
Thus, we suggest to phrase this problem as a hierarchical object detection problem:
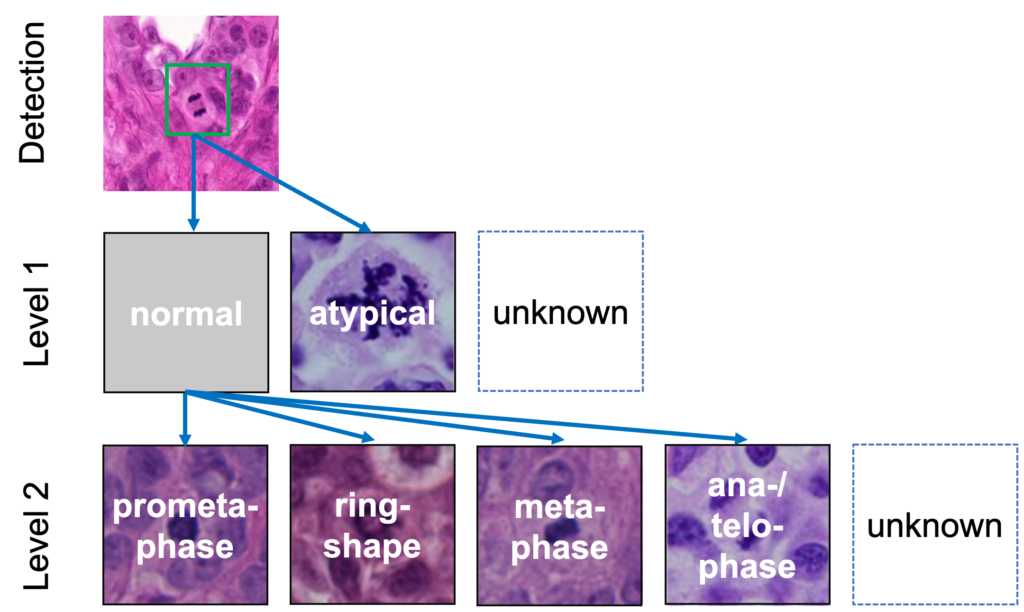
For this, we extended the fully convolutional single-state object detection (FCOS) approach by two subclassification heads:
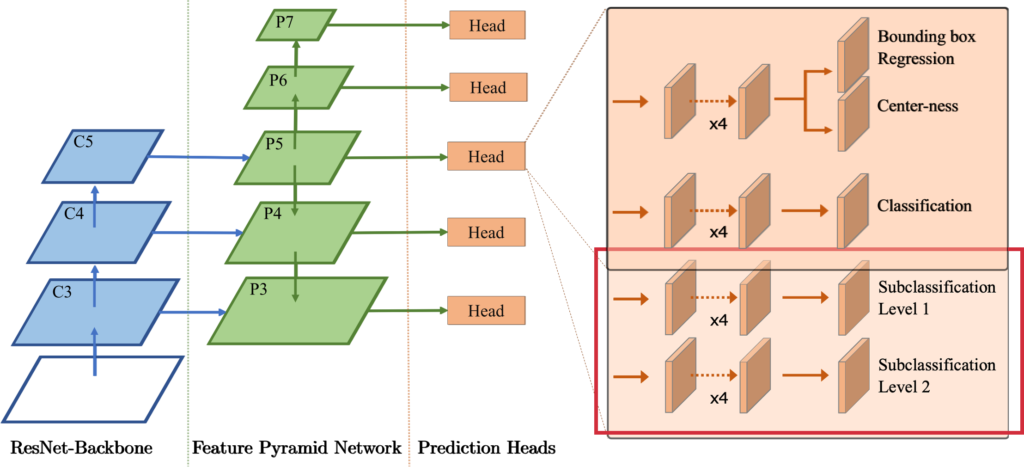
Our results indicate that this benefits both, the detection task, as well as the subclassification task, if we compare it to an FCOS baseline which discriminates 6 different classes of objects.
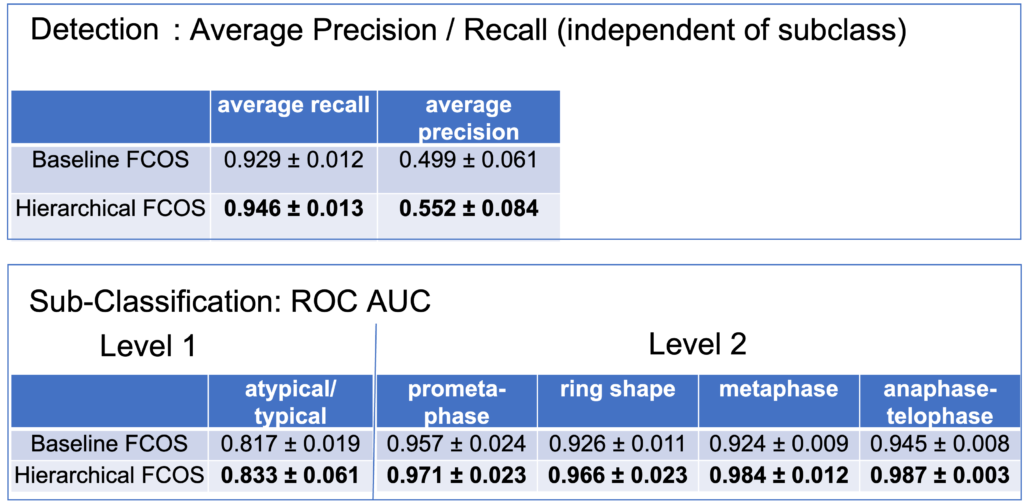
Link to the paper: https://doi.org/10.1007/978-3-658-41657-7_40
Talk on youtube:
No responses yet