Deep Learning-based Automatic Assessment of AgNOR-Scores, measuring proliferation speed [BVM 2023 best paper]
Assessing how fast cells divide
When assessing the malignancy of a tumor, we are interested in a couple of things. One of them is, how fast a tumor grows. In addition to the routinely used hematoxylin and eosin stain, there are many other more specialized stains used in pathology to assess specific biomarkers in tissue samples. One of these is the AgNOR immunohistochemical staining.
The „NOR“ in AgNOR comes from nucleolar organizer regions (NORs), which are parts of DNA involved in RNA transcription. When stained with a silver-based stain, the stained NORs are called AgNORs. The number of AgNORs per nucleus (i.e., the dark dots in the image above) is known to be correlated to cell growth and division speed (proliferation). More aggressive tumors have more numerous and smaller AgNORs (Webster et al.).
Assessing the AgNOR score, which is defined as the mean number of AgNORs in one hundred randomly selected nuclei, is a laborious task in routine pathology. Fortunately, this is also a pattern recognition task that can be solved very well using Deep Learning. In our work, we tried to solve the problem using an ensemble of five fully convolutional onestage object detectors (FCOS).
Because the evaluation of AgNOR from digital slides has not been extensively studied, and because we wanted to compare our algorithm with human raters, we also conducted a human-rater experiment with six pathology experts.
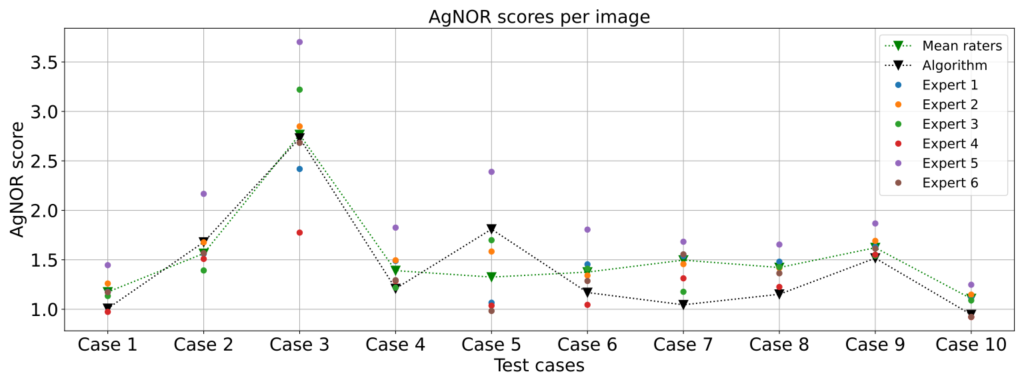
Insights from our study:
We found a couple of interesting things: First, our algorithm did quite well in scoring the AgNOR scores, as we found only a slight disagreement between the algorithm’s scores and the average expert scores (mean squared error: 0.054, mean absolute error was 0.201). We also found that the experts had some difficulty in arriving at consistent results. This may be because some of the AgNORs were placed at different focal lengths, and fine focusing (as shown in the animation below) was not possible, since commonly only a 2D image is acquired.
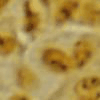
Overall, we were able to assess the AgNOR score with fairly human-like performance. Future work will focus on overcoming the problems mentioned above and using the results to evaluate the value of the AgNOR score as a prognostic factor for outcome prediction.
Link to the paper: https://link.springer.com/chapter/10.1007/978-3-658-41657-7_49
One response
[…] the best scientific contribution, congratulations! For a summary of the article, visit the joint blog of the AIMI & IMI […]