TL; DR: The MIDOG++ multi-domain mitotic figure dataset sets a new benchmark with 503 included cases across 7 domains with 12k annotations in total.
MIDOG++ – The largest multi-domain mitotic figure dataset
Data is said to be the new oil. While this statement is certainly a bit broad, accurate deep learning-based pattern recognition techniques would not be possible without the availability of high quantities of data. In particular in medical image recognition, what matters even more is the annotation quality of the images.
Mitotic figure detection is a standard task in the assessment of the malignancy of a tumor, and it is a well-known computer vision problem. Early datasets such as the MITOS12 were made publicly available and strongly contributed to the advancement of methods for mitotic figure recognition. However, these early data sets also lacked the data diversity that can be found in clinical applications, strongly limiting the generalization.
We identified the digitization device (whole slide scanner) to be a major influence in this diversity, leading to the inception of the MIDOG 2021 challenge. However, as MIDOG 2021 was limited to breast cancer, and there are a large number of other tumors where the mitotic count plays a role, the successor MIDOG 2022 included tissue from more tumor domains.
Today, we introduce MIDOG++ – an extended version of the MIDOG 2022 challenge dataset. With 7 domains (breast carcinoma, lung carcinoma, lymphosarcoma, neuroendocrine tumor, cutaneous mast cell tumor, cutaneous melanoma, and (sub)cutaneous soft tissue sarcoma), 503 cases, and approx. 12,000 annotations generated as a consensus between three experts, it is the largest multi-domain mitotic figure dataset to date.
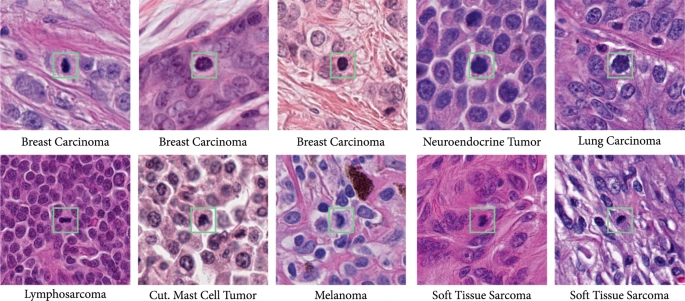
All details about the dataset and the baseline experiments can be found in the Scientific Data paper that we published alongside the dataset. We found that by using all domains, it is possible to train a pretty robust mitotic figure detector, which we tested on the independent 10-domain test set of the MIDOG 2022 challenge:
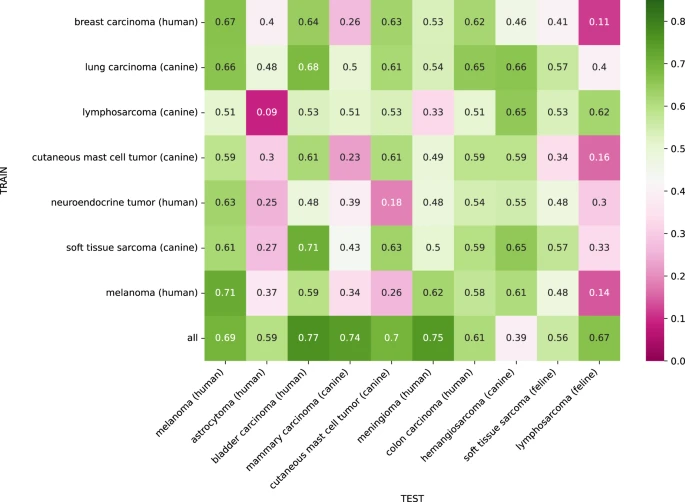
One response
[…] descriptor paper for a full description of the data set. To get a glimpse, we recommend reading the blog post about […]